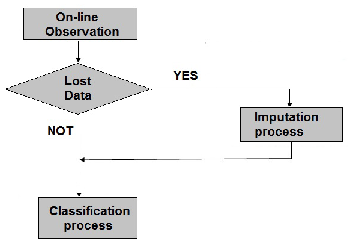 |
|
In this paper, the problem of fault diagnosis in complex industrial systems in the presence of missing data is addressed. Firstly, how to perform online imputation when there are missing values in the observations obtained by the data acquisition system is presented. Later, the possibility to apply advanced statistical techniques as Sequential Regression Multiple Imputation, Singular Value Decomposition, Local Least Squares Imputation and k- Nearest Neighbors as examples of possible tools to be used in the online imputation is displayed. In addition, the effects on the fault diagnosis process, when using these statistics tools to estimate the missing data are analyzed. A Neural Network Multi-layer Perceptron for the fault diagnosis system was used. The study was done using the Tennessee Eastman benchmark process. The results show the viability of the proposal.
Keywords: missing data, online imputation, statistical techniques, fault diagnosis, industrial process.
|
|
|